当前位置:网站首页>Toward a Unified Model
Toward a Unified Model
2022-08-11 06:16:00 【zhSunw】
Learning Multiple Adverse Weather Removal via Two-stage Knowledge Learning and Multi-contrastive Regularization: Toward a Unified Model
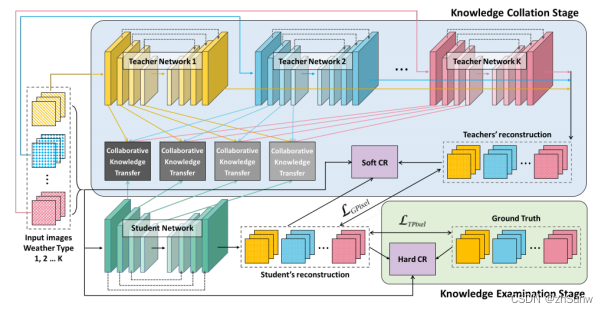
Two-stage Knowledge Learning (Collation-Examination) is designed on the basis of knowledge ledgedistillation and the method of contrastive learning is introduced.
Method
Collaborative Knowledge Transfer (CKT)
Progressive Feature Projector (PFP)
Project the features of the teacher and student networks into a common feature space, and calculate the L1 loss of the two projected features: Q represents the number of coding layers, Ti represents the ith teacher network, and S represents the student network
Projection is achieved by a small network consisting of several convolutional blocks with stride 1 and a ReLU activation function
Bidirectional Feature Matching
Project the projected features of the teacher network back to the original input space, and calculate the L1 loss of the two features to ensure the validity of the projection
Multi-contrastive Regularization (Two-stage Knowledge Learning)
Contrastive Regularization: v, v+, v- represent predicted samples, positive samples (target samples), and negative samples respectively; Ψ represents the features extracted by VGG-19; R is the number of negative samples.
Soft Contrastive Regularization. (Knowledge Collation (KC).)
Soft: Use teacher's predictions as positive samples to avoid the challenges of early learning
Hard Contrastive Regularization.(Knowledge Examination (KE).)
Hard: Use groundtruth as a positive sample to learn directly after training for a period of time
边栏推荐
猜你喜欢
第七届集美大学程序设计竞赛(个人赛)题解
LAGRANGIAN FLUID SIMULATION WITH CONTINUOUS CONVOLUTIONS
mysql基本概念之事务
CVPR2022——A VERSATILE MULTI-VIEW FRAMEWORK
梅科尔工作室-HarmonyOS应用开发第一次培训
梅科尔工作室-深度学习第二讲 BP神经网络
梅科尔工作室-华为云ModelArts第二次培训
SQL注入
The working principle and industry application of AI intelligent image recognition
Nodered系列—使用mqtt写入国产数据库tDengine
随机推荐
mAPH——Waymo数据集
DNS外带注入SQLMAP
架构设计杂谈
Rethinking LiDAR Object Detection in adverse weather conditions
《现代密码学》学习笔记——第五章 密钥分配与密钥管理
梅科尔工作室-DjangoWeb 应用框架+MySQL数据库第二次培训
Fragment 和 CardView
GBase 8s的多线程结构
【OAuth2】授权机制
GBase 8a技术特性-集群架构
梅科尔工作室-第四次PR培训笔记(字幕和标题动画,关键帧动画和声音处理)
如何修改严格模式让MySQL5.7插入用户表的方式新建用户成功?delete和drop的不同
azkaban集群部署
mysql基本概念之事务
【sqlyog】【mysql】csv导入问题
MGRE环境下的OSPF综合实验
软件架构之--MVC、MVP、MVVM
关于修改挂载到宿主机上的mysql配置文件不生效这件事
若依分离版—移动端开发通知公告功能
LAGRANGIAN FLUID SIMULATION WITH CONTINUOUS CONVOLUTIONS